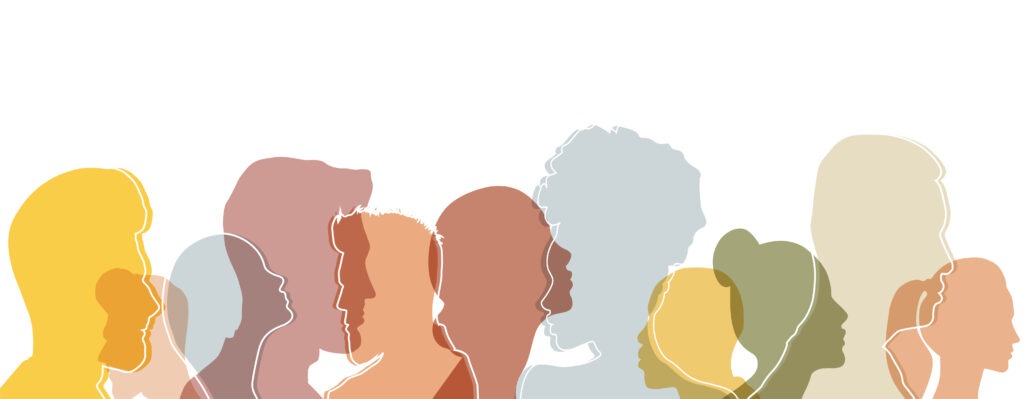
AI Personas Are Just Stereotyping in Disguise—Unless We Do Better
In the era of AI, machine learning, and large language models (LLMs), marketers are racing to build smarter, faster, more scalable ways to understand their audiences. One of the hottest trends right now? Using AI to generate personas or audience “clusters” that guide everything from creative briefs to campaign targeting.
But here’s the nuanced truth: AI-generated personas are often just advanced stereotyping unless they’re course-corrected intentionally.
Why This Happens: If The Data Is Shallow, So Is the Output
Most AI persona models are trained on massive datasets that reflect our collective digital footprint—search history, click behavior, sentiment analysis, browsing patterns, social media language. On the surface, it seems sophisticated. But under the hood, these models often reinforce lazy assumptions:
- If you’re a 35-year-old suburban mom, you must be driven by safety and family.
- If you follow left-leaning content, you’re emotional, empathetic, and communal.
- If you’ve purchased luxury goods, you’re aspirational and image-conscious.
Sure, these generalizations might correlate with statistical patterns. But correlation doesn’t equal causation—or understanding. AI clusters audiences based on patterns it sees, not the people beneath them. And too often, it codifies bias and obscures nuance.
Personas Aren’t the Problem—How We Build Them Is
The goal of audience segmentation has always been to better understand and serve people. But building personas based solely on surface-level traits (age, income, digital behaviors) or linguistic patterns extracted by LLMs runs the risk of flattening human complexity.
It becomes “automated profiling”—not meaningful insight. Real insight comes from digging into why people think, feel, and act the way they do:
- What are their cognitive tendencies?
- What emotional states drive their choices?
- What contextual or cultural forces are shaping their behavior?
This is where behavioral science, psycholinguistics, and AI can actually do something transformative—if used responsibly.
What Ethical, Effective AI Persona Modeling Should Look Like
To build personas that do more than stereotype, we need to start with psychology, not just data. Here’s a better approach:
- Begin with behavioral frameworks, such as Big 5 personality traits or motivational models (e.g., Maslow’s hierarchy, self-determination theory)
- Overlay psycholinguistic cues—using how people speak and write to infer emotional tone, decision-making frames, and attentional focus.
- Test for diversity within similarity—challenge your models to uncover cognitive and emotional diversity within demographic sameness.
- Build dynamic, fluid personas that evolve over time, channels, and context—not static archetypes frozen in a PowerPoint deck.
Done right, this moves us away from “Soccer Mom Sarah” and toward “Stability-Seeker Sarah who uses routines to manage uncertainty, but is open to new ideas when framed around trusted expertise.”
The Bottom Line: AI Is a Mirror—Not a Mind Reader
If we’re not careful, LLMs and AI will reflect the shallowest patterns we’ve already internalized, reinforcing the same stereotypes we’ve been trying to move past for years. Worse, because it’s generated by machines, it comes with a false sense of objectivity.
AI can be a powerful tool in human-centered marketing—but only if we combine it with human insight, behavioral nuance, and ethical rigor.